Demystifying Python Celery: key components and result storage
Imagine a seamless web experience where every click or interaction has a lightning-fast response. Behind the scenes, a powerful ally is at work — Python Celery. Asynchronous task queues, like Celery, are the unsung heroes of web development. They empower software programs to effortlessly delegate resource-intensive tasks to separate machines or processes, allowing your web application to stay responsive.
In this article, we embark on a journey to unravel the intricacies of task queues and dive deep into their architecture. Our primary focus will be on Celery, a household name among Python projects. However, the principles we'll explore here are not confined to Celery alone. Many other tools in the realm of web development follow similar patterns, making the knowledge gained here broadly applicable.
So, whether you're a seasoned developer looking to fine-tune your asynchronous task handling or a curious newcomer eager to grasp the magic behind smooth web interactions, keep reading! We're about to demystify the world of Python Celery and the art of task queue orchestration.
Exploring the Power of Asynchronous Task Queues
In broad terms, the reason for employing async task queues is the need for lightning-fast user responses. The most straightforward use case involves delegating time-consuming CPU-intensive tasks. However, the real star of the show is the ability to execute external API calls. When you rely on external services, the clock is no longer in your hands, and there's uncertainty about when, or even if, the results will arrive. System downtimes or glitches on the other end can keep you waiting indefinitely.
Another compelling use case for async tasks is in the realm of result value preparation and caching. They're your secret weapon when it comes to spreading out those massive database insertions over time, guarding against unintentional DDoS attacks on your own database. And let's not forget about cron jobs, which can be seamlessly managed using async tasks.
When it comes to wrangling async tasks in Python, you're spoiled for choice. While RQ is gaining some well-deserved attention lately, Celery remains the undisputed heavyweight champion. With its robust features and widespread adoption, Celery has long been the go-to tool for developers looking to harness the power of asynchronous task queues.
Read more: Dealing With Resource-Consuming Tasks on Celery
Understanding the Architecture of Async Task Queues
The challenge of running asynchronous tasks neatly aligns with the classic Producer/Consumer problem. Producers are responsible for depositing tasks into a queue, while consumers eagerly scan the beginning of the queue for pending tasks, select the first one, and execute it.

In the context of asynchronous task queues, these roles take on specific names. Producers are commonly represented by 'web nodes' or any system responsible for task submission. The queue itself plays the crucial role of the 'broker,' and the task executors are referred to as 'workers.' Interestingly, workers can not only process tasks but also add new tasks to the queue, effectively donning the hat of 'producers' themselves.
Now that we have a high-level overview, let's delve a bit deeper into the inner workings of this fascinating architecture.
Choosing The Ideal Celery Broker
The concept of a broker is straightforward—it's essentially a queue. However, the implementation of a queue in a computer system offers various options. One of the simplest methods is using a text file. Text files can hold a sequence of job descriptions to be executed, making them a potential choice for serving as the broker in our system. However, there's a caveat with text files; they are not designed to handle real application challenges like network communication and concurrent access. Consequently, for a more robust solution, we need to explore other options.
SQL databases, with their network capabilities and concurrent access handling, might seem like an attractive choice. However, they often fall short in terms of speed, making them less ideal for certain use cases. On the other end of the spectrum, NoSQL databases can be incredibly fast but may lack the level of reliability needed for critical tasks.
So, when it comes to building queues for asynchronous tasks, the goal is to strike a balance. You want a solution that is fast, reliable, and capable of handling concurrent operations. This is where tools like RabbitMQ, Redis, and SQS come into play.
Celery, being a versatile asynchronous task queue system, provides comprehensive support for both RabbitMQ and Redis. While SQS and Zookeeper are also available options, they are typically offered with limited capabilities. (For more information on these options, please refer here.)
In essence, the choice of your broker—the heart of your asynchronous task queue system—can significantly impact the performance and reliability of your application. It's a critical decision that can shape how your tasks are processed and executed, ensuring a seamless user experience.
Harmonizing Web and Worker Nodes in Celery
Web and worker nodes are essentially plain servers, with a key distinction: Web nodes handle incoming requests from the internet and dispatch jobs for asynchronous processing, while Workers are the machines responsible for picking up these tasks, executing them, and delivering responses. Despite this clear separation in functionality, it's common practice to house the code for both types of nodes within the same code repository. This practice, in fact, offers several advantages.
By co-locating the code for web and worker nodes, you create a synergy that allows them to reap the benefits of shared components such as models and services. This unified approach not only fosters code reusability but also serves as a bulwark against inconsistencies.
In the realm of asynchronous task queues, this approach ensures a smoother workflow and enhances the overall efficiency of your system. Join us as we explore the merits of this unified approach in optimizing the performance and maintainability of your web application.
Handling Tasks in the Celery Framework
Here is an example showcasing the execution of tasks using Celery:
Worker Node:
Web Node:
In the first block, the code represents a task that should run asynchronously. It is implemented in the worker node. The second block showcases the code that places a job in the queue to be executed. This code is typically found in the web node. In this specific example, the web node places an "add" job and waits until the result becomes available. Upon receiving the response, the result is printed.
Let's explore a Django-specific example:
Worker Node:
Web Node:
In this case, the web node places a task to update the number of attendees for an event. It is important to note that passing complex objects, like a [.code-inline] Model[.code-inline] instance, as task parameters can lead to problems.
When objects are serialized and stored in the broker, security vulnerabilities may arise if complex objects are allowed. Additionally, database objects passed as parameters can become outdated during the time period between the task placement and its execution.
To tackle these issues, it is recommended to pass the ID of the object as a parameter and fetch a fresh copy from the database within the task implementation. Here's an updated version of the example:
By following this approach, you ensure that the task works with the most up-to-date version of the object.
Storing and Retrieving Task Results in Celery
In the previous examples, we have treated the [.code-inline]delay[.code-inline] and [.code-inline]get[.code-inline] functions as a single action, but in reality, they serve distinct purposes. The [.code-inline]delay[.code-inline] function places the task in the queue and returns a promise that allows monitoring of the task's status and retrieval of its result once it's ready. On the other hand, calling [.code-inline]get[.code-inline] on this promise blocks the execution until the result becomes available. However, we have missed an essential piece of the architecture by not mentioning the results backend.
Introducing the Results Backend
The results backend plays a crucial role in storing task results. Typically, you can utilize the same instance you are using for the broker to act as the [.code-inline]results backend[.code-inline].
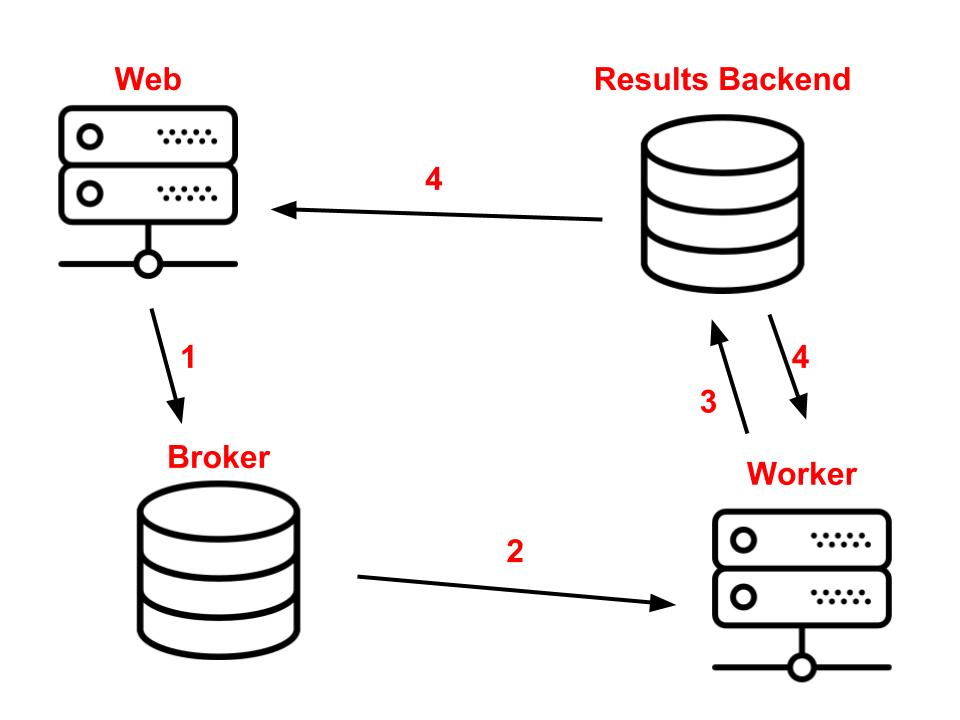
While there are other technologies available beyond the supported broker options that can serve as the results backend in Celery, the implementation details may differ based on the chosen technology. For instance, when using Postgres, the [.code-inline]get[.code-inline] method performs polling to check if the result is ready, while technologies like Redis handle this through a pub/sub protocol.
By comprehending the basics of the components in the Celery architecture, you now have a stronger understanding of how it works, gaining confidence in utilizing this powerful tool.
If you found this post helpful and are eager to expand your knowledge about Celery, I recommend taking a look at my other blog post: Celery in the Wild: Tips and Tricks for Running Async Tasks in the Real World.
Feel free to reach out if you have any further questions or need additional assistance. Let's explore the exciting possibilities together!